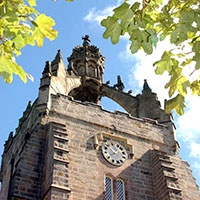
MEng, AMIChemE
Research PG
- About
-
- Email Address
- l.boston.19@abdn.ac.uk
- Office Address
Room 201, Fraser Noble Building
School of Engineering
King's College
Aberdeen
AB24 3UE
- School/Department
- Combined Studies
- Research
-
Research Overview
Computational fluid dynamics; lattice Boltzmann method; optimisation; mixing; anaerobic digestion
Research Areas
Engineering
Research Specialisms
- Bioengineering
- Chemical Engineering
- Computational Physics
- Fluid Mechanics
- Mathematical Modelling
Our research specialisms are based on the Higher Education Classification of Subjects (HECoS) which is HESA open data, published under the Creative Commons Attribution 4.0 International licence.
Current Research
Project Title: In Silico Optimisation of Viscous Mixing of Shear-Thinning Liquids
Project Summary: Mixing condition optimisation is vital within anaerobic digesters to ensure a suitable balance between the competing objectives of minimising power consumption and maximising mixing quality. Existing relevant optimisations tend to focus on methane production at low solids concentrations (≈ 1 %), using traditional computational fluid dynamics solvers such as the finite volume and element methods, and take a one-variable-at-a-time approach to helical ribbon impeller design. The main aim of this project is to employ a systematic design of experiments optimisation framework with an efficient Lattice Boltzmann Method hydrodynamic solver to allow efficient multi-objective optimisation of mixing systems, with a target application of high-solids anaerobic digester design for sustainable chemical production. The solver and framework are to be tested and validated to prove their applicability.
The optimisation framework consists of four stages: 1. Data screening using a definitive screening design to determine the most significant effects of input parameters on output responses. 2. Using an efficient in-house LBM solver to generate simulation data for training polynomial regression equations à la response surface methodology via a forward stepwise regression approach utilising the corrected Akaike's Information Criterion. 3. Validating regression equations against additional simulations. 4. Optimising a composite desirability function using the reduced gradient algorithm of Minitab® statistical software using the regression equations as surrogate models.
This framework is used to solve the multi-objective optimisation problem of minimising the impeller power number, percentage of dead volumes, and maximum shear rate within a mixing tank stirred by a helical ribbon impeller containing a non-Newtonian, shear-thinning, power-law liquid, for a range of flow behaviour indices, by altering impeller and tank geometry. Surrogate models are found to replicate simulation results with a deviation of 3 % to 18 %. This framework is also tested against an empirical correlation-based method, wherein the framework demonstrates greater flexibility. The power number predicted by the correlation method deviates by ≤ 24 % compared to simulations, while the surrogate model deviates by ≤ 8 %.
- Teaching
-
Teaching Responsibilities
2024/25
EX4030: Separation Processes 2 - Lab and tutorial demonstrator
2023/24
EG501V: Computational Fluid Dynamics - Lab demonstrator
2022/23
EG501V: Computational Fluid Dynamics - Lab demonstrator
2021/22
EG501V: Computational Fluid Dynamics - Lab demonstrator
2020/21
EG501V: Computational Fluid Dynamics - Lab demonstrator
EX4530: Separation Processes 2 - Lab demonstrator
2019/20
EG501V: Computational Fluid Dynamics - Lab demonstrator
EX4530: Separation Processes 2 - Lab demonstrator
Non-course Teaching Responsibilities
2021/22
Supervised MEng research project